The rapid rise of generative artificial intelligence like OpenAI’s GPT-4 has brought remarkable advancements, but it also presents significant risks.
One of the most pressing issues is model collapse, a phenomenon where AI models trained on largely AI-generated content tend to degrade over time. This degradation occurs as AI models lose information about their true underlying data distribution, resulting in increasingly similar and less diverse outputs full of biases and errors.
As the internet becomes flooded with real-time AI-generated content, the scarcity of new, human-generated or natural data further exacerbates this problem. Without a steady influx of diverse, high-quality data, AI systems risk becoming less accurate and reliable.
Read more:
Researchers warn we could run out of data to train AI by 2026. What then?
Amid these challenges, synthetic data has emerged as a promising solution. Designed to closely mimic the statistical properties of real-world data, it can provide the necessary volume for training AI models while ensuring the inclusion of diverse data points.
(Shutterstock)
Synthetic data does not contain any real or personal information. Instead, computer algorithms draw on statistical patterns and characteristics observed in real datasets to generate synthetic ones. These synthetic datasets are tailored to researchers’ specific needs, offering scalable and cost-effective alternatives to traditional data collection.
My research explores the advantages of synthetic data in creating more diverse and secure AI models, potentially addressing the risks of model collapse. I also probe key challenges and ethical considerations in the future development of synthetic data.
Uses of synthetic data
From training AI models and testing software to ensuring privacy in data sharing, artificially generated information that replicates the characteristics of real-world data has wide-ranging applications.
Synthetic data in healthcare helps researchers analyze patient trends and health outcomes, supporting the development of advanced diagnostic tools and treatment plans. This data is produced by algorithms that replicate real patient data while incorporating diverse and representative samples during the data generation process.
In finance, synthetic data is used to model financial scenarios and predict market trends while safeguarding sensitive information. It also allows institutions to simulate critical financial events, enhancing stress testing, risk management and compliance with regulatory standards.
Synthetic data also supports the development of responsive and accurate AI-driven customer service support systems. By training AI models on datasets that replicate real interactions, companies can improve service quality, address diverse customer inquiries and enhance support efficiency — all while maintaining data integrity.
Across various industries, synthetic data helps manage the dangers of model collapse. By providing new datasets to supplement or replace human-generated data, it reduces logistical challenges associated with data cleaning and labelling, raising standards for data privacy and integrity.
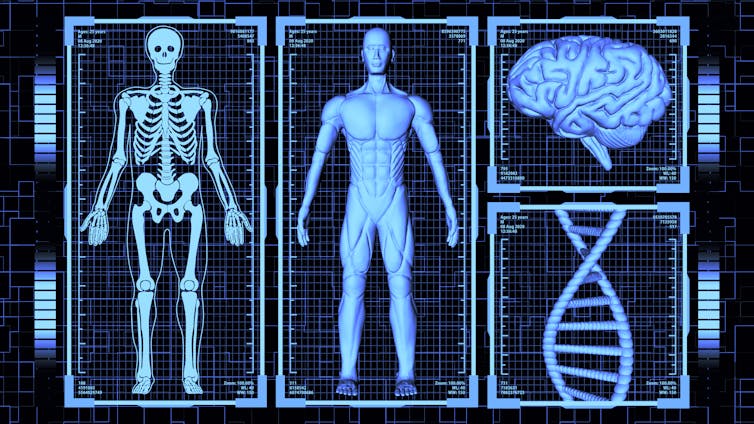
(Shutterstock)
Dangers of synthetic data
Despite its many benefits, synthetic data presents several ethical and technical challenges.
A major challenge is ensuring the quality of synthetic data, which is determined by its ability to accurately reflect the statistical properties of real data while maintaining privacy. High-quality synthetic data is designed to enhance privacy by adding random noise to the dataset.
Yet this noise can be reverse-engineered, posing a significant privacy threat as highlighted in a recent study by United Nations University.
Reverse-engineered synthetic data runs the risk of de-anonymization. This occurs when synthetic datasets are deconstructed to reveal sensitive personal information. This is particularly relevant under regulations like the European Union’s General Data Protection Regulation (GDPR), which applies to any data that can be linked back to an individual. Although programming safeguards can mitigate this risk, reverse engineering cannot be entirely eliminated.
Synthetic data can also introduce or reinforce biases in AI models. While it can reliably generate diverse datasets, it still struggles to capture rare but critical nuances present in real-world data.
If the original data contains biases, these can be replicated and amplified in the synthetic data, leading to unfair and discriminatory outcomes. This issue is particularly concerning in sectors like healthcare and finance, where biased AI models can have serious consequences.
Synthetic data also struggles to capture the full spectrum of human emotions and interactions, resulting in less effective AI models. This limitation is especially relevant in emotion-AI applications, where understanding emotional nuances is critical for accurate and empathetic responses. For example, while synthetic data generalizes common emotional expressions, it can overlook subtle cultural differences and context-specific emotional cues.
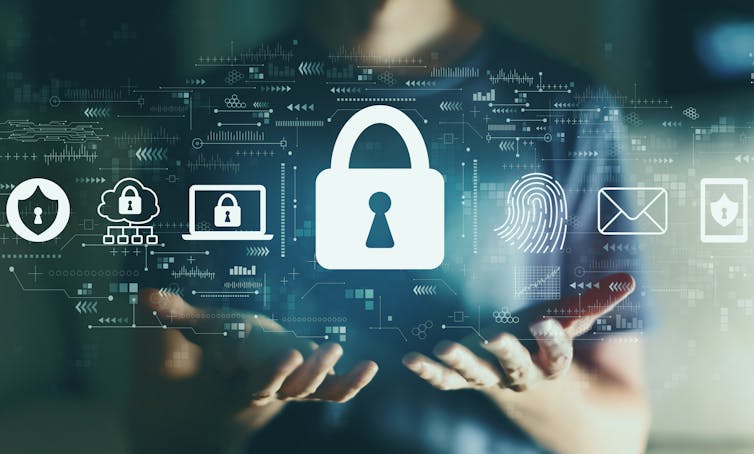
(Shutterstock)
Advancing AI
Understanding the differences between artificially generated data and data from human interactions is crucial. In the coming years, organizations with access to human-generated data will have a significant advantage in creating high-quality AI models.
While synthetic data offers solutions to privacy and data availability challenges that can lead to model collapse, over-reliance on it can recreate the very issues it seeks to solve. Clear guidelines and standards are needed for its responsible use.
This includes robust security measures to prevent reverse engineering and ensuring datasets are free from biases. The AI industry must also address the ethical implications of data sourcing and adopt fair labour practices.
There is an urgent need to move beyond categorizing data as either personal or non-personal. This traditional dichotomy fails to capture the complexity and nuances of modern data practices, especially in the context of synthetic data.
As synthetic data incorporates patterns and characteristics from real-world datasets, it challenges binary classifications and requires a more nuanced approach to data regulation. This shift could lead to more effective data protection standards aligned with the realities of modern AI technologies.
By managing synthetic data use and addressing its challenges, we can ensure that AI advances while maintaining accuracy, diversity and ethical standards.
Source link
lol