arXiv:2407.07221v1 Announce Type: new
Abstract: Poisoning attacks compromise the training phase of federated learning (FL) such that the learned global model misclassifies attacker-chosen inputs called target inputs. Existing defenses mainly focus on protecting the training phase of FL such that the learnt global model is poison free. However, these defenses often achieve limited effectiveness when the clients’ local training data is highly non-iid or the number of malicious clients is large, as confirmed in our experiments. In this work, we propose FLForensics, the first poison-forensics method for FL. FLForensics complements existing training-phase defenses. In particular, when training-phase defenses fail and a poisoned global model is deployed, FLForensics aims to trace back the malicious clients that performed the poisoning attack after a misclassified target input is identified. We theoretically show that FLForensics can accurately distinguish between benign and malicious clients under a formal definition of poisoning attack. Moreover, we empirically show the effectiveness of FLForensics at tracing back both existing and adaptive poisoning attacks on five benchmark datasets.
Source link
lol
Tracing Back the Malicious Clients in Poisoning Attacks to Federated Learning
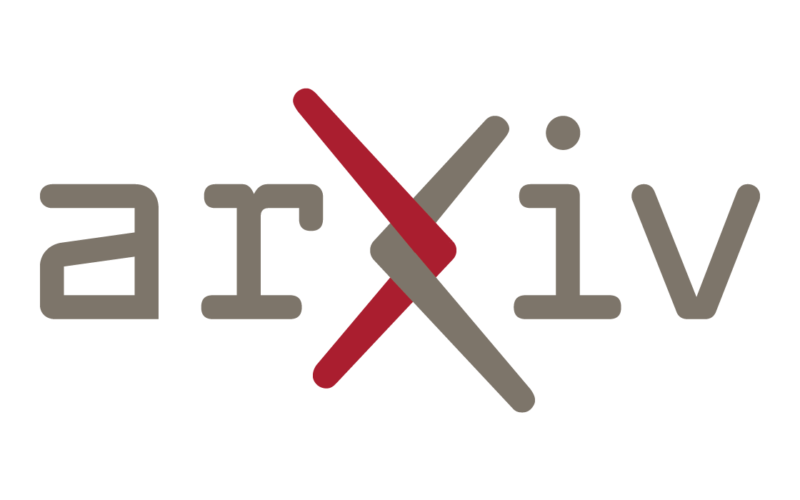