arXiv:2407.06503v1 Announce Type: new
Abstract: In this paper, we investigate preference-based reinforcement learning (PbRL) that allows reinforcement learning (RL) agents to learn from human feedback. This is particularly valuable when defining a fine-grain reward function is not feasible. However, this approach is inefficient and impractical for promoting deep exploration in hard-exploration tasks with long horizons and sparse rewards. To tackle this issue, we introduce LOPE: Learning Online with trajectory Preference guidancE, an end-to-end preference-guided RL framework that enhances exploration efficiency in hard-exploration tasks. Our intuition is that LOPE directly adjusts the focus of online exploration by considering human feedback as guidance, avoiding learning a separate reward model from preferences. Specifically, LOPE includes a two-step sequential policy optimization process consisting of trust-region-based policy improvement and preference guidance steps. We reformulate preference guidance as a novel trajectory-wise state marginal matching problem that minimizes the maximum mean discrepancy distance between the preferred trajectories and the learned policy. Furthermore, we provide a theoretical analysis to characterize the performance improvement bound and evaluate the LOPE’s effectiveness. When assessed in various challenging hard-exploration environments, LOPE outperforms several state-of-the-art methods regarding convergence rate and overall performance. The code used in this study is available at url{https://github.com/buaawgj/LOPE}.
Source link
lol
Preference-Guided Reinforcement Learning for Efficient Exploration
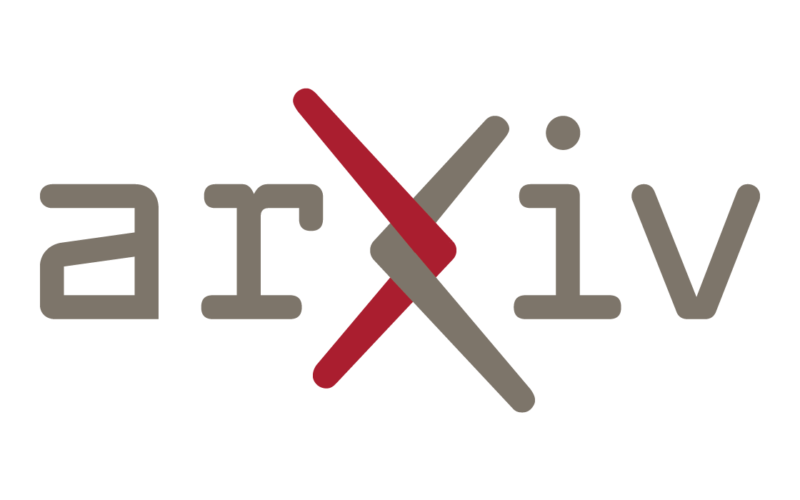