arXiv:2407.03545v1 Announce Type: new
Abstract: Is explainability a false promise? This debate has emerged from the insufficient evidence that explanations aid people in situations they are introduced for. More human-centered, application-grounded evaluations of explanations are needed to settle this. Yet, with no established guidelines for such studies in NLP, researchers accustomed to standardized proxy evaluations must discover appropriate measurements, tasks, datasets, and sensible models for human-AI teams in their studies.
To help with this, we first review fitting existing metrics. We then establish requirements for datasets to be suitable for application-grounded evaluations. Among over 50 datasets available for explainability research in NLP, we find that 4 meet our criteria. By finetuning Flan-T5-3B, we demonstrate the importance of reassessing the state of the art to form and study human-AI teams. Finally, we present the exemplar studies of human-AI decision-making for one of the identified suitable tasks — verifying the correctness of a legal claim given a contract.
Source link
lol
On Evaluating Explanation Utility for Human-AI Decision Making in NLP
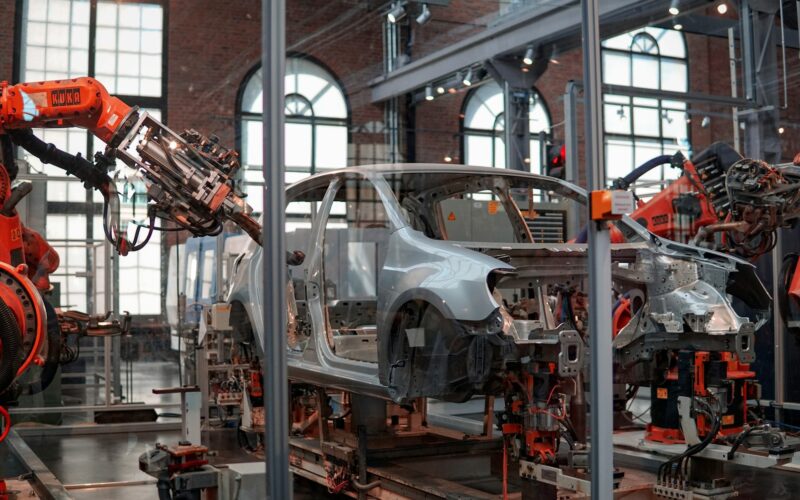