arXiv:2407.03425v1 Announce Type: new
Abstract: Autonomous mobile robots deployed in urban environments must be context-aware, i.e., able to distinguish between different semantic entities, and robust to occlusions. Current approaches like semantic scene completion (SSC) require pre-enumerating the set of classes and costly human annotations, while representation learning methods relax these assumptions but are not robust to occlusions and learn representations tailored towards auxiliary tasks. To address these limitations, we propose LSMap, a method that lifts masks from visual foundation models to predict a continuous, open-set semantic and elevation-aware representation in bird’s eye view (BEV) for the entire scene, including regions underneath dynamic entities and in occluded areas. Our model only requires a single RGBD image, does not require human labels, and operates in real time. We quantitatively demonstrate our approach outperforms existing models trained from scratch on semantic and elevation scene completion tasks with finetuning. Furthermore, we show that our pre-trained representation outperforms existing visual foundation models at unsupervised semantic scene completion. We evaluate our approach using CODa, a large-scale, real-world urban robot dataset. Supplementary visualizations, code, data, and pre-trained models, will be publicly available soon.
Source link
lol
Lift, Splat, Map: Lifting Foundation Masks for Label-Free Semantic Scene Completion
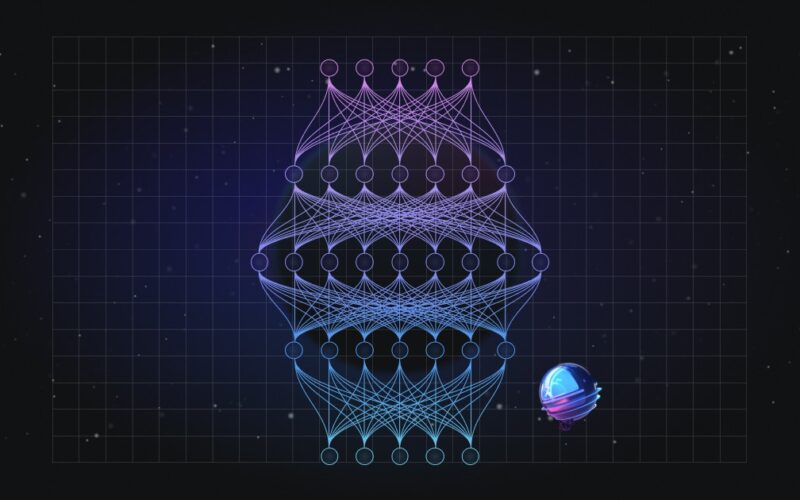