arXiv:2407.02641v1 Announce Type: new
Abstract: Multi-variate time series forecasting is an important problem with a wide range of applications. Recent works model the relations between time-series as graphs and have shown that propagating information over the relation graph can improve time series forecasting. However, in many cases, relational information is not available or is noisy and reliable. Moreover, most works ignore the underlying uncertainty of time-series both for structure learning and deriving the forecasts resulting in the structure not capturing the uncertainty resulting in forecast distributions with poor uncertainty estimates. We tackle this challenge and introduce STOIC, that leverages stochastic correlations between time-series to learn underlying structure between time-series and to provide well-calibrated and accurate forecasts. Over a wide-range of benchmark datasets STOIC provides around 16% more accurate and 14% better-calibrated forecasts.
STOIC also shows better adaptation to noise in data during inference and captures important and useful relational information in various benchmarks.
Source link
lol
Learning Graph Structures and Uncertainty for Accurate and Calibrated Time-series Forecasting
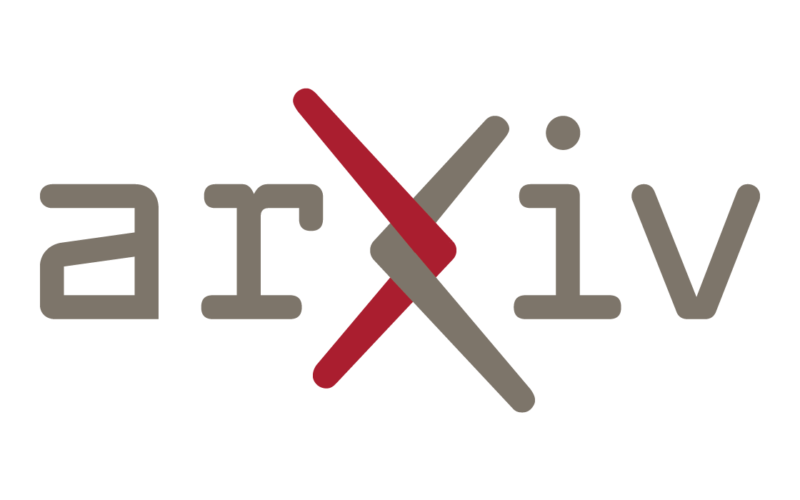