arXiv:2407.02536v1 Announce Type: new
Abstract: Given a set emph{S} of spatial feature types, its feature instances, a study area, and a neighbor relationship, the goal is to find pairs $$ such that emph{C} is a statistically significant regional-colocation pattern in $r_{g}$. This problem is important for applications in various domains including ecology, economics, and sociology. The problem is computationally challenging due to the exponential number of regional colocation patterns and candidate regions. Previously, we proposed a miner cite{10.1145/3557989.3566158} that finds statistically significant regional colocation patterns. However, the numerous simultaneous statistical inferences raise the risk of false discoveries (also known as the multiple comparisons problem) and carry a high computational cost. We propose a novel algorithm, namely, multiple comparisons regional colocation miner (MultComp-RCM) which uses a Bonferroni correction. Theoretical analysis, experimental evaluation, and case study results show that the proposed method reduces both the false discovery rate and computational cost.
Source link
lol
Reducing False Discoveries in Statistically-Significant Regional-Colocation Mining: A Summary of Results
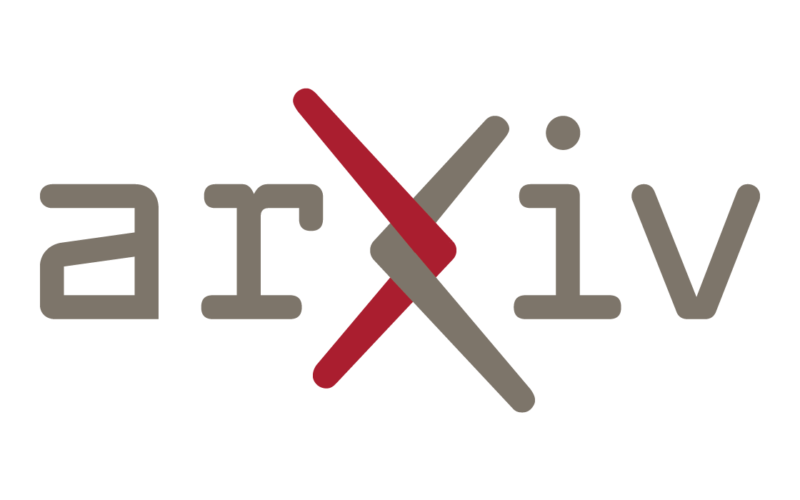